by Nick Ballou, Oxford Internet Institute
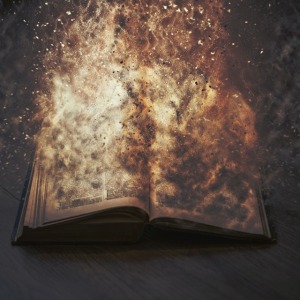
Scientific fraud is worryingly common, though rarely talked about. It has been happening for years, but now Artificial Intelligence programs could supercharge it. If they do that could undermine Science itself.
Investigators of scientific fraud have found that large numbers of researchers have manipulated their results, invented data, or even produced nonsensical papers in the hope that no one will look closely enough to notice. Often, no one does. The problem is that science is built on the foundation of all the research that has gone before. If we can no longer trust that past research is legitimate, the whole system of science begins to break down. AI has the potential to supercharge this process.
We’re not at that point yet, luckily. But there are concerning signs that generative AI systems like ChatGPT and DALLE-E might bring us closer. By using AI technology, producing fraudulent research has never been easier, faster, or more convincing. To understand, let’s first look at how scientific fraud has been done in the past.
How fraud happens
Until recently, fraudsters would need to go through some difficult steps to get a fraudulent research paper published. A typical example might look like this:
Step 1: invent a title
Fraudsters look for a popular but very broad research topic. We’ll take an example of a group of fraudsters known as the Tadpole Paper Mill. They published papers about cellular biology. To choose a new paper to create, the group would essentially use a simple generator, or algorithm, based on a template. This uses a simple technique first used by Christopher Strachey to write love letters in an early “creative” program in the 1950s.
For each “hole” in the template a word is chosen from a word list.
- Pick the name of a molecule
- Either a protein name, a drug name or an RNA molecule name
- eg mir-488
- Pick a verb
- From alleviates, attenuates, exerts, …
- eg inhibits
- Pick one or two cellular processes
- From invasion, migration, proliferation, …
- eg cell growth and metastasis
- Pick a cancer or cell type
- From lung cancer, ovarian cancer, …
- eg renal cell carcinoma
- Pick a connector word
- From by, via, through, …
- eg by
- Pick a verb
- From activating, targeting, …
- eg targeting
- Pick a name
- Either a pathway, protein or miRNA molecule name
- eg hMgn5
This produces a complicated-sounding title such as “mir-488 inhibits cell growth and metastasis in renal cell carcinoma by targeting hMgn5”. This is the name of a real fraudulent paper created this way.
Step 2: write the paper
Next, the fraudsters create the text of the paper. To do this, they often just plagiarise and lightly edit previous similar papers, substituting key words in from their invented title perhaps. To try to hide the plagiarism, they automatically swap out words, replacing them with synonyms. This often leads to ridiculous (and kind of hilarious) replacements, like these found in plagiarised papers:
- “Big data” –> “Colossal information”
- “Cloud computing” –> “Haze figuring”
- “Developing countries” –> “Creating nations”
- “Kidney failure” –> “Kidney disappointment”
Step 3: add in the results
Lastly, the fraudsters need to create results for the fake study. These usually appear in papers in the form of images and graphs. To do this, the fraudsters take the results from several previous papers and recombine them into something that looks mostly real, but is just a Frankenstein mess of other results that have nothing to do with the current paper.
A new paper is born
Using that simple formula, fraudsters have produced thousands of fabricated articles in the last 10 years. Even after a vast amount of effort, the dedicated volunteers who are trying to clean up the mess have only caught a handful.
However, committing fraud like this successfully isn’t exactly easy, either: the fraudsters still need to come up with a research idea, write the paper themselves without copying too much from previous research, and make up results that look convincing—at least at first glance.
AI: Adding fuel to the fire
So what happens when we add modern generative AI programs into the mix? They are Artificial Intelligence programs like ChatGPT or DALL-E that can create text or pictures for you based on written requests.
Well, the quality of the fraud goes up, and the difficulty of producing it goes way down. This is true for both text and images.
Let’s start with text. Just now, I asked ChatGPT-4 to “write the first two paragraphs of a research paper on a cutting edge topic in psychology.” I then asked it to “write a fake results table that shows a positive relationship between climate change severity and anxiety”. I won’t copy the whole thing—in part because I encourage you to try this yourself to see how it works (not to actually create a fake paper!)—but here’s a sample of what it came up with:
“As the planet faces increasing temperatures, extreme weather events, and environmental degradation, the mental health repercussions for populations worldwide become a crucial area of investigation. Understanding these effects is vital for developing strategies to support communities in coping with the psychological challenges posed by a changing climate.”
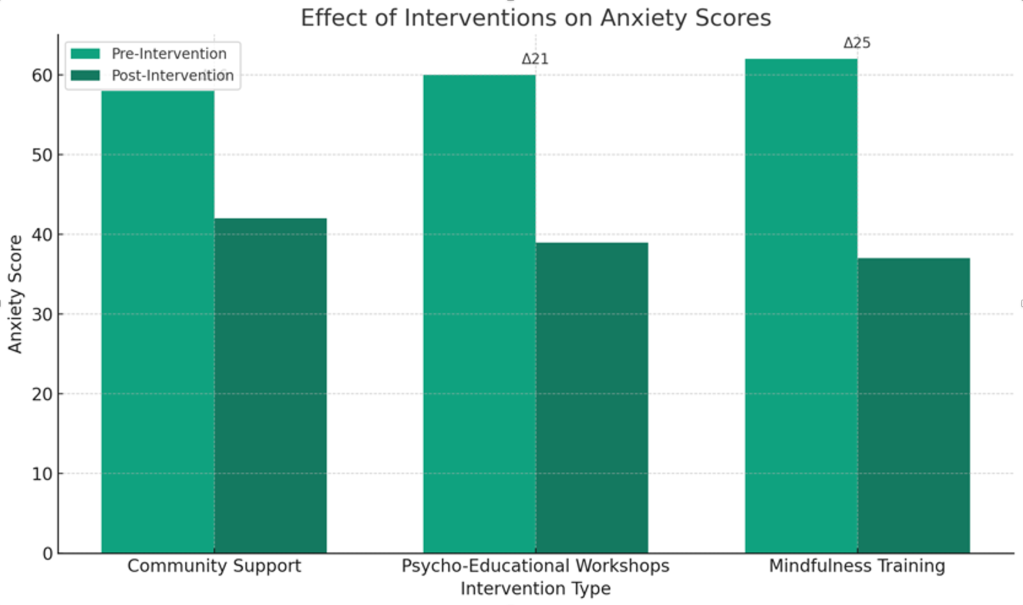
As someone who has written many psychology research papers, I would find these results very difficult to identify as AI-generated—it looks and sounds very similar to how people in my field write, and it even generated Python code to analyse the fake data. I’d need to take a really close look at the origin of the data and so on to figure out that it’s fraudulent.
But that’s a lot of work required from me as a fraud-buster. For the fraudster, doing this takes about 1 minute, and would not be detected by any plagiarism software in the way previous kinds of fraud can be. In fact, this might only be detected if the fraudsters make a sloppy mistake, like leaving in a disclaimer from the model as in the paper caught below!
Generative AIs are not close to human intelligence, at least not yet. So, why are they so good at producing convincing scientific research, something that’s commonly seen as one of the most difficult things humans can do? Two reasons play a big part: (1) scientific research is very structured, and (2) there’s a lot of training data. In any given field of research, most papers tend to look pretty similar—an introduction section, a method describing what the researchers did, a results section with a few tables and figures, and a discussion that links it back to the wider research field. Many journals even require a fixed structure. Generative AI programs work using Machine Learning – they learn from data and the more data they are given the better they become. Give a machine learning program millions of images of cats, telling it that is what they are, and it can become very good at recognising cats. Give it millions of images of dogs and it will be able to recognise dogs too. With roughly 3 million scientific papers published every year, generative AI systems are really good at taking these many, many examples of what a scientific report looks like, and producing similar sounding, and similarly structured pieces of text. They do it by predicting what word, sentence and paragraph would be good to come next based on probabilities calculated from all those examples.
Trusting future research
Most research can still be trusted, and the vast majority of scientists are working as hard as they can to advance human knowledge. Nonetheless, we all need to look carefully at research studies to ensure that they are legitimate, and we should be on extra alert as generative AI becomes even more powerful and widespread. We also need to think about how to improve universities and research culture generally, so that people don’t feel like they need to commit scientific fraud—something that usually happens because people are desperate to get or keep a job, or be seen as successful and reap the rewards. Somehow we need to change the game so that fraud no longer pays.
What do you think? Do you have ideas for how we can prevent fraud from happening in the first place, and how can we better detect it when it does occur? It is certainly an important new research topic. Find a solution and you could do massive good. If we don’t find solutions then we could lose the most successful tool human-kind has ever invented that makes all our lives better.
Related Magazines …
More on …
EPSRC supports this blog through research grant EP/W033615/1,