by Paul Curzon, Queen Mary University of London
Computer science research in part involves inventing new algorithms or improving new ones. But what does that mean. Let’s explore some mazes to explore algorithms.
What does computer science research involve? It is very varied: from interviewing people to find out what the real problems that need solving in their lives or jobs are; to running experiments to find out what works and what doesn’t; to writing programs to solve problems.
Improving algorithms
A core part of much research is coming up with new and better algorithms that solve particular problems. The kind of algorithm could be anything from a new more secure cryptographic protocol, or a better way to rank the results of a search engine, to a new more effective machine learning algorithm that is less likely to make things up, or perhaps can better explain how it came to its conclusions.
What does it mean to come up with a better algorithm though? Once a problem is solved, isn’t it solved? Let’s explore a simple problem to see. Let’s explore mazes. Solve the simple maze puzzle above before you go on. Find a route that gets the mouse to the cheese.
Wandering around mazes, finding algorithms
If you’ve ever been in a hedge maze in the garden of some stately home, or a corn field maze, the chances are you just dived in and wandered rather aimlessly. Perhaps you tried to remember which way you went at each junction, to avoid going down the same dead-ends more than once. How about solving the paper version of a maze puzzle like the one above? Now perhaps you looked ahead to spot dead-ends to avoid tracing wrong paths with your pencil.
Probably what you are doing is at least a little random. You could, in theory at least, end up going back over the same paths, never taking the right one and and never getting to the middle. Could we come up with an algorithm that guarantees to solve mazes? To be an algorithm it would need to guarantee you ended up finding a path to the centre of the maze if you followed the steps of the algorithm precisely. It should also work for any maze, or at least all mazes of a particular kind. Ideally, the algorithm gives you a path that can then be followed by anyone without them having to run the algorithm themselves. They can just follow the path generated by the algorithm for that maze.
Wall-following
In fact lots of maze algorithms have been invented. Perhaps the one most people have heard of, if they know of any maze algorithm, is called Wall-following. It is very simple to do, You just pick a wall at the entrance either to the left or right and then follow it, If in a garden maze, keep your hand on the hedge as you walk round. If doing a paper puzzle, draw the path sticking to the chosen wall. Try it on the following simple maze.
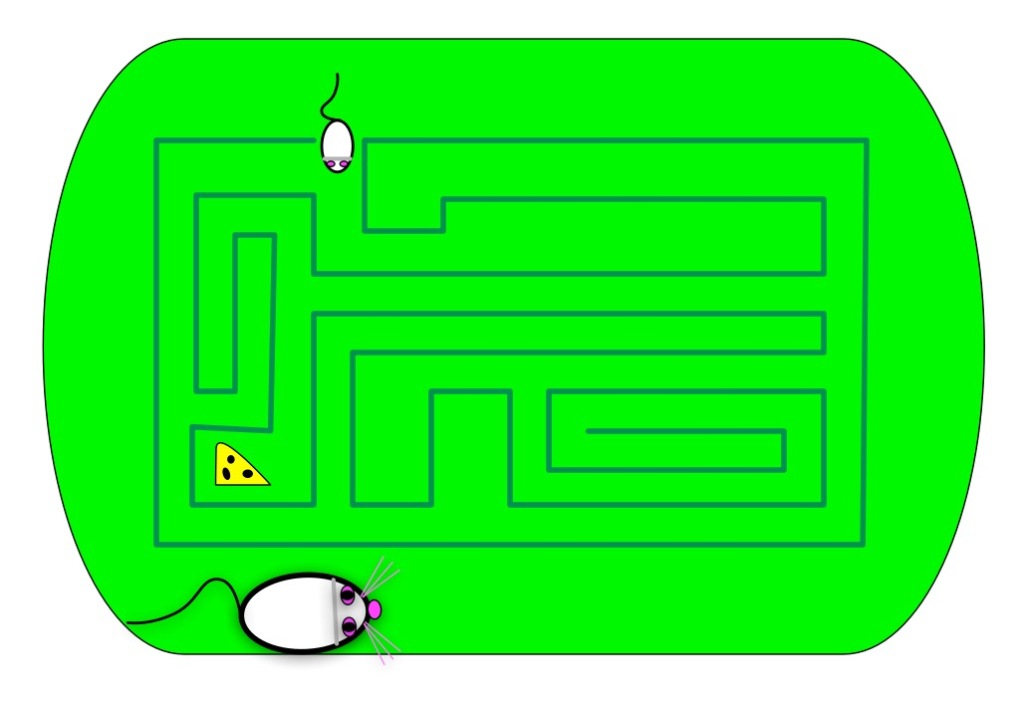
Simply connected
The wall-following algorithm will guarantee to get you to the centre of the maze, and back out again too, but only as long as the maze is what is called simply connected. That just means the maze is constructed from a single hedge (or one unbroken drawn line) not a series of unconnected hedges. If you look at both examples above you will see I created them by just drawing a single wiggly line.
If a maze is simply connected then it cannot have looping paths, so no going round in circles for ever. It will also only have one entrance/exit. That shows the first aspect of inventing algorithms that is important. They often only work for some situations, not all. You must be sure you know what situations they do and don’t work.
Often the earliest algorithms invented to solve a problem are like wall-following: they only work for simple situations. Other people then come along and find new algorithms that can cover more problems (here more mazes). Can you tweak the wall-following maze algorithm to work even if there are multiple exits from the maze, for example? As it stands our algorithm could just take you from the entrance straight out of another exit without exploring much of the maze at all! See the end for one simple way to tweak the algorithm. What if there are paths that take you round in circles? Can you come up with an algorithm to deal with that?
Some times the improvements invented just involve tweaking an existing algorithm as with dealing with multiple exits in a maze. Some times a whole new algorithm is needed.
Faster, higher, stronger?
Even for a simple constrained version of the problem, like simply connected mazes, people can invent better algorithms. What does better mean for a maze? Well one way you might have a better algorithm is if it is faster in coming up with a solution. Another is that the solution it comes up with is faster. For a maze that means a shorter (ideally the shortest) path to the centre. Wall following may get you in to the centre (and out again) but you probably will have discovered a very long path that takes you in and out of lots of dead-ends needlessly. You do find a path to the centre, but it may be a very long path. Can you come up with an algorithm that finds shorter paths?
We will explore an algorithm that does next.
More to come…
Some solutions
The result of wall following on our simple maze
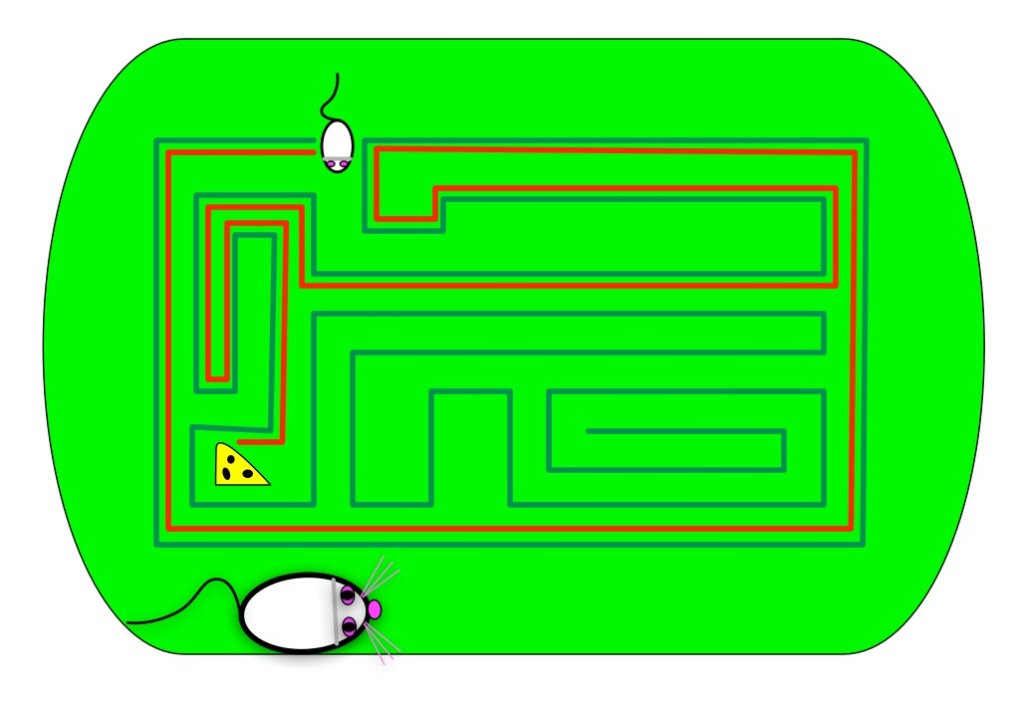
One way to deal with multiple exits
To deal with a maze that has multiple exits, so multiple breaks in the outer wall, tweak the wall-following algorithm as follows. First mark the exit you use to enter the maze, so you know when you return to it. If you come to any other exit then pretend there is a gate there and keep following the wall as though it were unbroken and there were no exit.
More on …
- Mousemat maze puzzles [on Teaching London Computing]
Magazines …
EPSRC supports this blog through research grant EP/W033615/1.